LLM Course
The LLM course is divided into three parts: 🧩 LLM Fundamentals is optional and covers fundamental knowledge about mathematics, Python, and neural networks. 🧑🔬 The LLM Scientist focuses on building the best possible LLMs using the latest techniques. 👷 The LLM Engineer focuses on creating LLM-based applications and deploying them.
Coder EvalPlus
EvalPlus is a rigorous evaluation framework for LLM4Code, with: ✨ HumanEval+: 80x more tests than the original HumanEval! ✨ MBPP+: 35x more tests than the original MBPP! ✨ EvalPerf: evaluating the efficiency of LLM-generated code! ✨ Framework: our packages/images/tools can easily and safely evaluate LLMs on above benchmarks.
Speculative Decoding in vLLM
Speculative decoding in vLLM is a powerful technique that accelerates token generation by leveraging both small and large models in tandem. In this blog, we’ll break down speculative decoding in vLLM, how it works, and the performance improvements it brings. This content is based on a session from our bi-weekly vLLM Office Hours, where we discuss techniques and updates to optimize vLLM performance.
vLLM v0.6
vLLM achieves 2.7x higher throughput and 5x faster TPOT (time per output token) on Llama 8B model, and 1.8x higher throughput and 2x less TPOT on Llama 70B model. A month ago, we released our performance roadmap committing to performance as our top priority. We will start by diagnosing the performance bottleneck in vLLM previously. Then we will describe the solution we implemented and landed in the past month. Finally, we will showcase the benchmarks of the latest vLLM release v0.6.0 other inference engines.
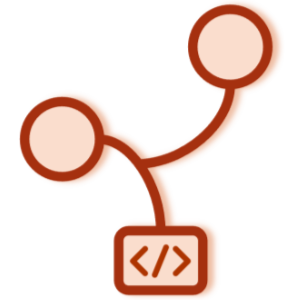
SGLang v0.3
We’re excited to announce the release of SGLang v0.3, which brings significant performance enhancements and expanded support for novel model architectures. Here are the key updates: Up to 7x higher throughput for DeepSeek Multi-head Latent Attention (MLA). Up to 1.5x lower latency with torch.compile on small batch sizes. Support for interleaved text and multi-image/video in LLaVA-OneVision. Support for interleaved window attention and 2x longer context length in Gemma-2.
Qwen2-VL
Qwen2-VL is the latest version of the vision language models in the Qwen model families. SoTA understanding of images of various resolution & ratio: Qwen2-VL achieves state-of-the-art performance on visual understanding benchmarks, including MathVista, DocVQA, RealWorldQA, MTVQA, etc. Understanding videos of 20min+: with the online streaming capabilities, Qwen2-VL can understand videos over 20 minutes for high-quality video-based question answering, dialog, content creation, etc.
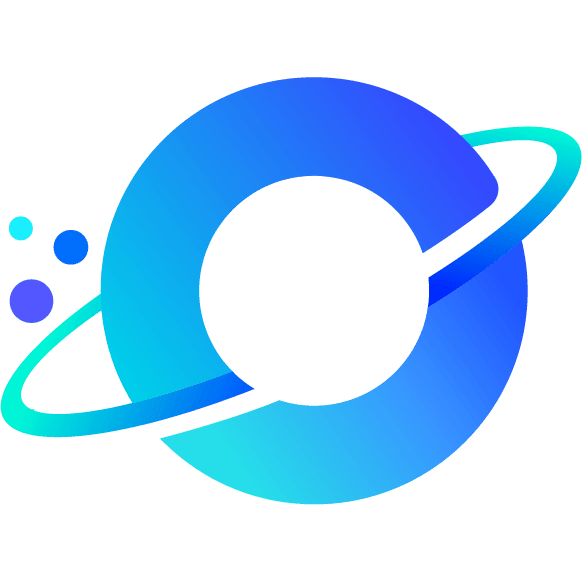
Text2SQL Leaderboard
Text-to-SQL (or Text2SQL), as the name implies, is to convert text into SQL. A more academic definition is to convert natural language problems in the database field into structured query languages that can be executed in relational databases. Therefore, Text-to-SQL can also be abbreviated as NL2SQL. Input: natural language questions, such as Query the relevant information of the table t_user, and the results are sorted in descending order by id. Output: SQL, such as SELECT * FROM t_user ORDER BY id DESC.
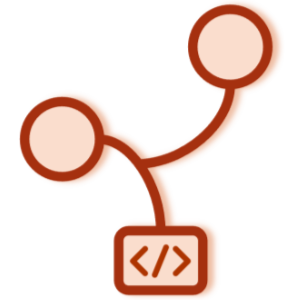
SGLang v0.2
Through our operational experiences and in-depth research, we’ve continuously enhanced the underlying serving systems for the Chatbot Arena platform, spanning from the high-level multi-model serving framework, FastChat, to the efficient serving engine for LLMs and VLMs, SGLang Runtime (SRT). While existing options like TensorRT-LLM, vLLM, MLC-LLM, and Hugging Face TGI have their merits, we found them sometimes hard to use, difficult to customize, or lacking in performance. This motivated us to develop SGLang v0.2, aiming to create a serving engine that is not only user-friendly and easily modifiable but also delivers top-tier performance.