Chatbot Arena
This leaderboard is based on the following three benchmarks. Chatbot Arena - a crowdsourced, randomized battle platform for large language models (LLMs). We use 1.1M+ user votes to compute Elo ratings. MT-Bench - a set of challenging multi-turn questions. We use GPT-4 to grade model responses. MMLU (5-shot) - a test to measure a model’s multitask accuracy on 57 tasks.
DeepSeek-V2
We introduce DeepSeek-V2, a strong Mixture-of-Experts (MoE) language model characterized by economical training and efficient inference. It comprises 236B total parameters, of which 21B are activated for each token. Compared with DeepSeek 67B, DeepSeek-V2 achieves stronger performance, and meanwhile saves 42.5% of training costs, reduces the KV cache by 93.3%, and boosts the maximum generation throughput to more than 5 times.
Coder EvalPlus
EvalPlus is a rigorous evaluation framework for LLM4Code, with: ✨ HumanEval+: 80x more tests than the original HumanEval! ✨ MBPP+: 35x more tests than the original MBPP! ✨ Evaluation framework: our packages/images/tools can easily and safely evaluate LLMs on above benchmarks. File a request to add your models on our leaderboard!
Llama 3
Today, we’re excited to share the first two models of the next generation of Llama, Meta Llama 3, available for broad use. This release features pretrained and instruction-fine-tuned language models with 8B and 70B parameters that can support a broad range of use cases. This next generation of Llama demonstrates state-of-the-art performance on a wide range of industry benchmarks and offers new capabilities, including improved reasoning.
LLM Course
The LLM course is divided into three parts: 🧩 LLM Fundamentals covers essential knowledge about mathematics, Python, and neural networks. 🧑🔬 The LLM Scientist focuses on building the best possible LLMs using the latest techniques. 👷 The LLM Engineer focuses on creating LLM-based applications and deploying them. For an interactive version of this course, I created two LLM assistants that will answer questions and test your knowledge in a personalized way:

Qwen 1.5
With Qwen1.5, we are open-sourcing base and chat models across six sizes: 0.5B, 1.8B, 4B, 7B, 14B, 32B, 72B, and an MoE model. In line with tradition, we’re also providing quantized models, including Int4 and Int8 GPTQ models, as well as AWQ and GGUF quantized models. To enhance the developer experience, we’ve merged Qwen’s code into Hugging Face transformers.
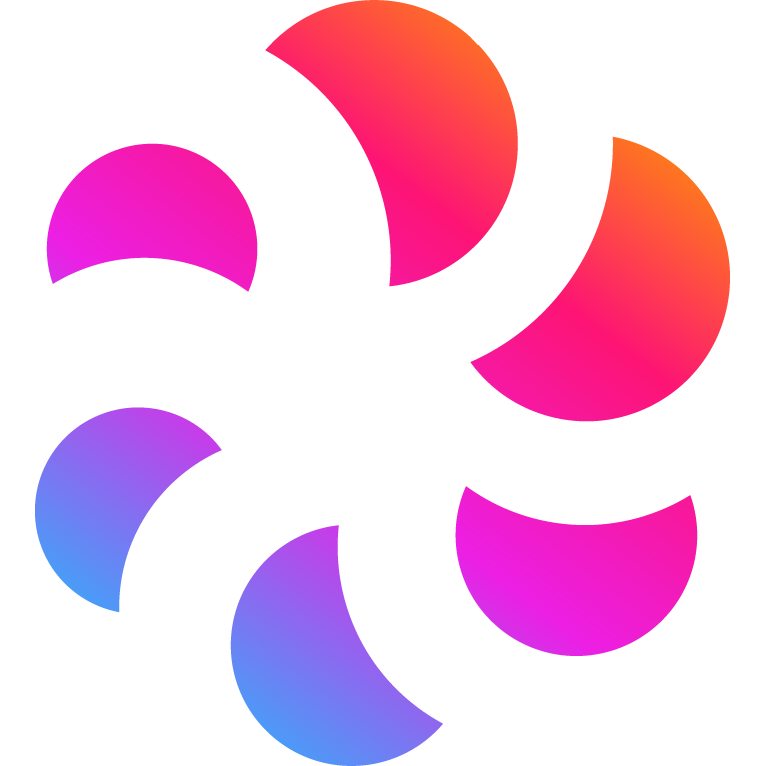
Accelerating Self-Attentions for LLM Serving with FlashInfer
LLM (Large Language Models) Serving quickly became an important workload. The efficacy of operators within Transformers – namely GEMM, Self-Attention, GEMV, and elementwise computations are critical to the overall performance of LLM serving. While optimization efforts have extensively targeted GEMM and GEMV, there is a lack of performance studies focused on Self-Attention in the context of LLM serving.
DeepSpeed-FastGen Performance and Feature Enhancements
DeepSpeed-FastGen is an inference system framework that enables easy, fast, and affordable inference for large language models (LLMs). From general chat models to document summarization, and from autonomous driving to copilots at every layer of the software stack, the demand to deploy and serve these models at scale has skyrocketed. DeepSpeed-FastGen utilizes the Dynamic SplitFuse technique to tackle the unique challenges of serving these applications and offer higher effective throughput than other state-of-the-art systems.